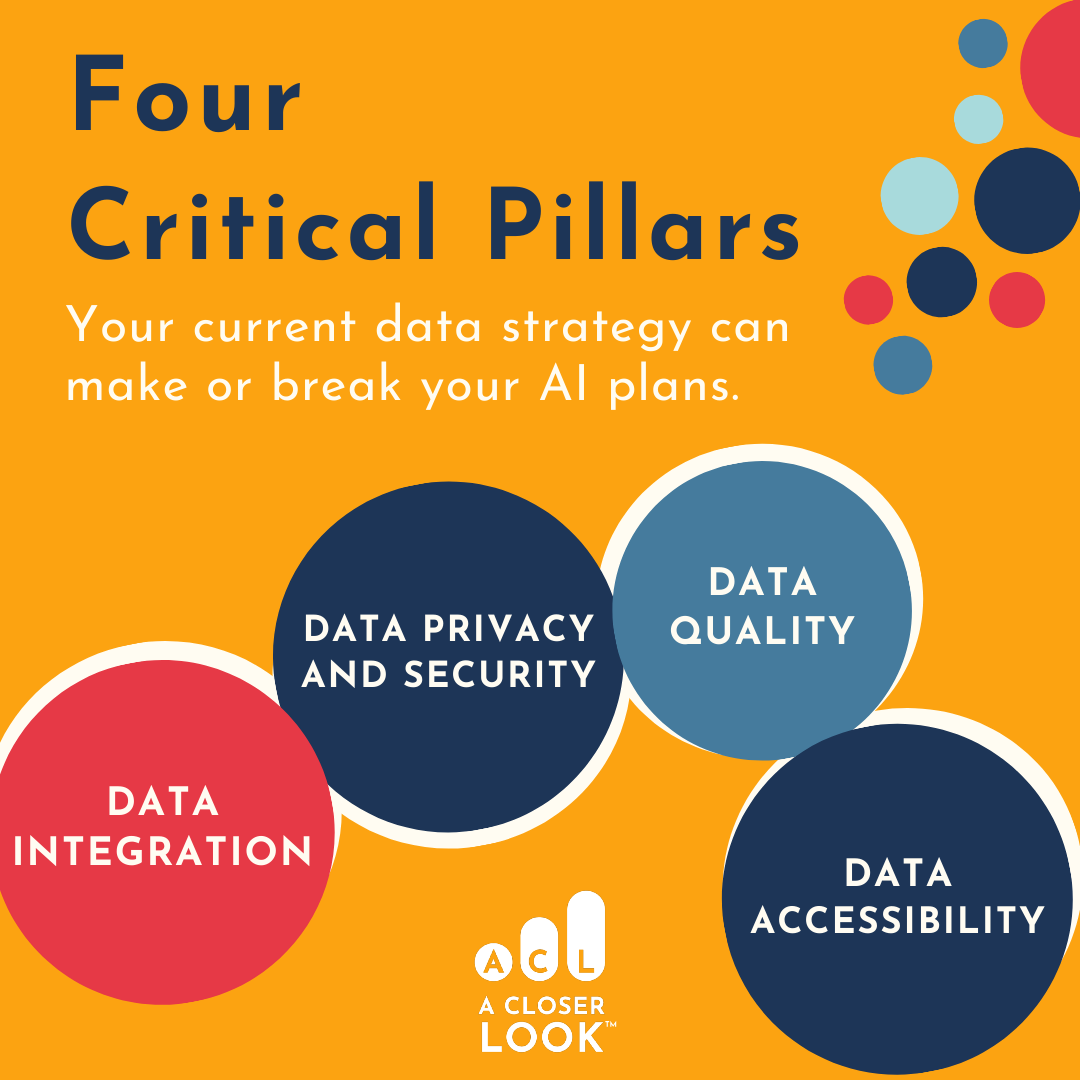
Is Your Current Data Strategy Setting You Up for AI Success?
I continue to speak with organizations that ask the question: “𝑯𝒐𝒘 𝒊𝒎𝒑𝒐𝒓𝒕𝒂𝒏𝒕 𝒊𝒔 𝒐𝒖𝒓 𝒄𝒖𝒓𝒓𝒆𝒏𝒕 𝒅𝒂𝒕𝒂 𝒔𝒕𝒓𝒂𝒕𝒆𝒈𝒚 𝒘𝒉𝒆𝒏 𝒕𝒉𝒊𝒏𝒌𝒊𝒏𝒈 𝒂𝒃𝒐𝒖𝒕 𝑨𝑰 𝒊𝒎𝒑𝒍𝒆𝒎𝒆𝒏𝒕𝒂𝒕𝒊𝒐𝒏?”
My answer is always the same: it is CRITICAL.
Have you heard the saying, “𝑮𝒂𝒓𝒃𝒂𝒈𝒆 𝒊𝒏, 𝒈𝒂𝒓𝒃𝒂𝒈𝒆 𝒐𝒖𝒕?”
The hard reality is that many companies are nowhere near ready for generative AI implementation because they lack an effective data strategy.
Before an organization can consider AI implementation, they should review their current data strategy in four key areas:
1. Data Quality
High-quality data is the foundation of effective AI systems. A robust data strategy ensures that the data used for AI training is accurate, relevant, and reliable. It involves data collection, cleansing, normalization, and ensuring data completeness. Without clean and reliable data, AI models may produce inaccurate or biased results, leading to poor decision-making.
2. Data Accessibility
AI systems require access to the right data at the right time. A data strategy should include mechanisms for data storage, organization, and retrieval. It should define data governance policies, access controls, and data sharing mechanisms to ensure that relevant data is available for AI models when needed. Accessibility to diverse and comprehensive datasets enables AI algorithms to learn and generalize effectively.
3. Data Integration
Many organizations have data spread across various systems and departments. A data strategy should consider how to integrate and aggregate data from multiple sources to create a unified and comprehensive view. Integration challenges, such as data format inconsistencies (this is a major problem for many companies) and data silos, need to be addressed to ensure that AI models can access the relevant information.
4. Data Privacy and Security
A robust data strategy should address privacy and security concerns associated with sensitive data. It should comply with applicable data protection regulations and ensure that data is handled and stored securely. Without strong data privacy and security measures, AI implementation can lead to breaches, legal issues, and the erosion of customer trust.
It is also important to remember that AI systems continuously learn and improve over time. A data strategy should include mechanisms for iterative data collection, feedback loops, and model retraining. It should allow for monitoring AI performance, capturing user feedback, and incorporating new data to enhance the accuracy and effectiveness of AI models.
Conducting a thorough and comprehensive assessment of your current data strategy will go a long way toward saving time and money and reducing frustration when you’re ready to implement your generative AI program.